
Tencent's quiet collaboration with DeepSeek enhances AI model performance
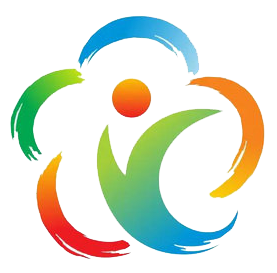
by Lu Keyan
In a move that underscores China’s growing strength in open-source AI development, Tencent has quietly partnered with DeepSeek to enhance the performance of DeepEP, a communication library central to the training of large AI models. The collaboration, which only came to light recently via a GitHub post by a DeepSeek engineer, reflects a rare yet significant cooperation between two of the country’s leading AI players.
According to the engineer, Tencent’s contributions brought about a “huge speedup” in DeepEP’s capabilities, directly benefiting all developers using DeepSeek’s open-source offerings.
Jiemian News spoke exclusively with Tencent’s StarLake Network team, the group behind the infrastructure powering its proprietary Hunyuan model, to learn more about the collaboration.
The technical exchange dates back to February this year, when DeepSeek open-sourced five core codebases aimed at allowing developers to reproduce high-performance training with a fraction of the hardware traditionally required. Among them was DeepEP—a library designed for communication within Mixture-of-Experts (MoE) models, which are used to reduce the cost and computational burden of training and deploying large-scale models such as GPT-4 and DeepSeek itself.
Tencent was an early adopter of the MoE framework in China, having implemented it in Hunyuan by early 2024. Previously, such models relied on Nvidia’s proprietary NCCL communication library, which posed high costs and limited flexibility. DeepEP offered a more accessible alternative, but its performance was uneven—particularly in the RoCE (RDMA over Converged Ethernet) networks commonly used by Chinese tech firms. Designed initially for InfiniBand, DeepEP struggled to maintain speed and efficiency on RoCE, leading to significant communication delays during model training.
These delays had a tangible cost. Dr. Xia Yinben, chief architect of the StarLake Network Lab, explained that inefficient networking forces expensive GPUs to idle while waiting for data transfers, leading to higher operational costs and slower responses for users.
Tencent’s advantage in addressing this issue, Xia said, stemmed from its long-running investments in networking technologies driven by high-demand applications across QQ, WeChat, online gaming, and cloud services. In 2022, the company began developing a dedicated network architecture tailored to AI workloads, known as StarLake.
The team optimized DeepEP’s performance under RoCE by adapting it to Tencent’s in-house TRMT (Tencent Remote Memory Transport) communication library. Drawing on research into the RoCEv2 protocol stack and dual-port network interface cards, they sought to better utilize available bandwidth while reducing latency. TRMT enabled GPUs to bypass the CPU and directly manage RDMA (Remote Direct Memory Access), minimizing control overhead and accelerating data exchange.
Tencent reports that these enhancements led to a 100% performance improvement under RoCEv2 and a 30% gain in InfiniBand environments. In practical terms, said Huang Xiaojie, one of the network architects involved, “a 10% performance gain in training translates to a 10% cost saving. For inference, it also means users wait less—say, from 10 seconds to 9 seconds per query.” While those gains remain internally benchmarked and may vary under different workloads or hardware conditions, they suggest meaningful efficiency improvements in both model training and deployment.
Tencent’s emphasis on RoCE over InfiniBand reflects broader strategic considerations. InfiniBand, favored in high-performance computing for its low latency, is largely dominated by Nvidia and carries higher costs and supply-chain risks. From the outset, Tencent built its AI infrastructure around Ethernet-based RoCE and developed its own communication libraries, first TCCL and more recently TRMT.
Chen Mingzhuo, another architect from the StarLake team, said Tencent and DeepSeek maintained ongoing communication not only around troubleshooting but also on the future evolution of AI networking. Their shared priority is minimizing GPU idle time caused by communication bottlenecks.
Traditionally, data transfer coordination within AI systems has relied on the CPU. Tencent’s approach is to link multiple GPUs more tightly, allowing them to access each other’s memory directly. This architecture reduces the need for CPU mediation and compensates for the lower compute capacity of domestic GPUs—an increasingly common constraint in China’s AI ecosystem.
The optimized version of DeepEP has since been contributed back to the open-source community and deployed in Tencent’s Hunyuan model. Other Chinese tech firms have also expressed interest in the enhancements and provided feedback, signaling a broader impact on the domestic AI infrastructure landscape.
Tencent, in this case, is both a beneficiary and contributor to the DeepSeek ecosystem. During Tencent’s recent earnings call, chairman and CEO Pony Ma expressed admiration for DeepSeek’s openness and efficiency, calling it “a truly open and free product” and noting that Tencent’s cloud services and its AI assistant Yuanbao have both integrated DeepSeek models.
The collaboration also reflects a deeper commitment by Tencent to open-source participation. Beyond cost efficiency or technical convenience, the company sees open-source development as key to building trust and accelerating innovation in an increasingly competitive global AI race.